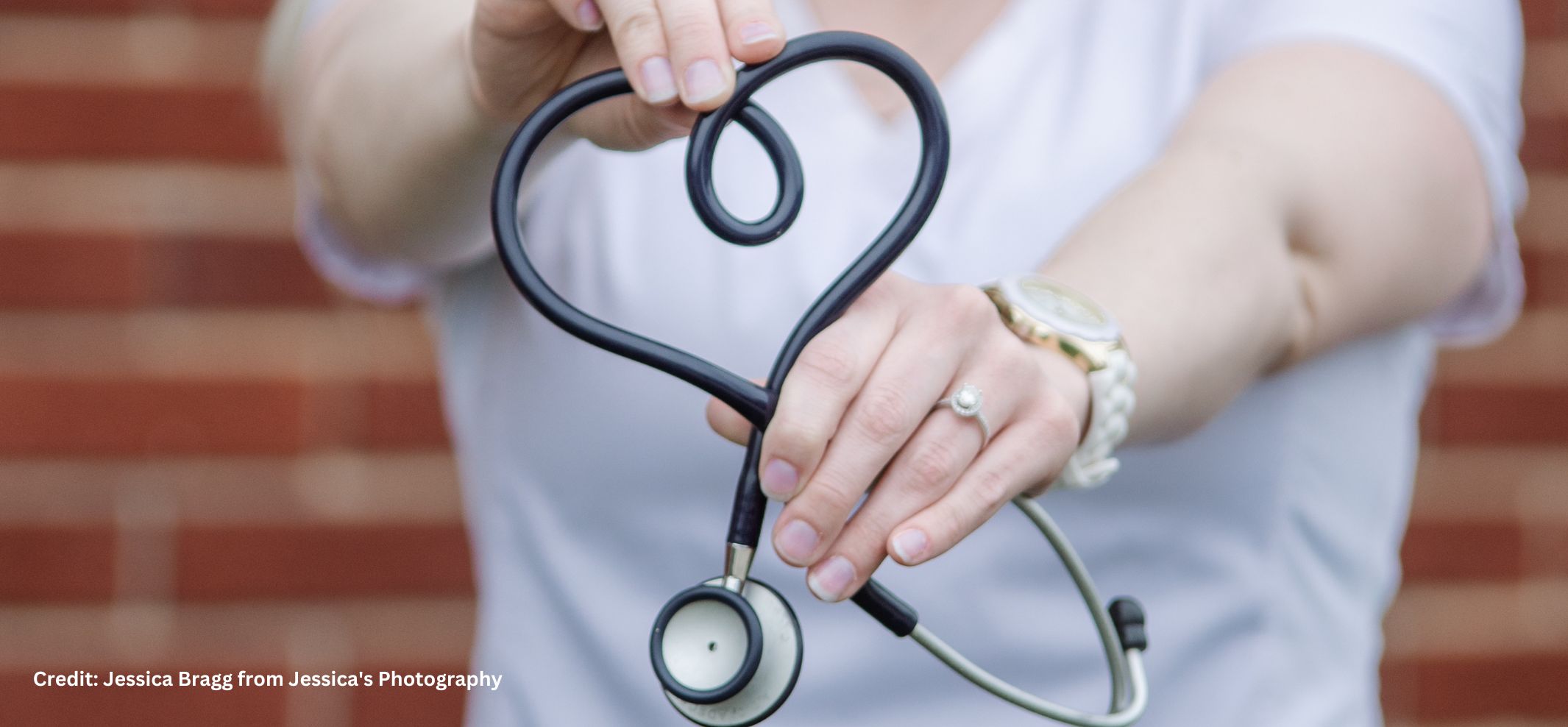
A care management system must be evaluated in three areas: comprehensiveness, inclusivity, and analytics focus. Care management programs apply systems, science, incentives, and information to improve medical. practice and assist consumers and their support system to become engaged in a collaborative process. designed to manage medical/social/mental health conditions more effectively. The goal of care.
Care management’s overarching goal is to improve patient health. To get there, the model also aims to improve care coordination, reduce hospital visits, and boost patient engagement.
First, a care management system must be exactly that: a system. Electronic medical records (EMR) or care coordination alone cannot meet the needs of healthcare organizations under value-based care. A true system brings a suite of products that integrate data from all sources, stratify patient risk, organize patient intake, manage patients through comprehensive care coordination, present two-way communication between patients and care managers, and measure care team performance.
What’s needed is a suite of tools with features in all five core competencies of care management:
These are the tools that comprise a comprehensive care management system, but two other areas more acutely define its value and effectiveness.
A care management system should not be bound by a single EMR and should span a variety of other data sources. This enables communication across the entire care team, which can comprise patients, acute care, primary care, specialty care, therapists, social workers, and care managers. When there are multiple health systems and employers working together in a wellness program, the variety of data sources and care team members grows even more complex. Therefore, a care management platform must sit at a level higher than an individual EMR. It must pull data from every system and be the platform where communication takes place.
The care management system should have analytics in its DNA, which means the system’s developers should also have analytics in their DNA. The system must be able to analyze data to figure out trends and patterns that drive better outcomes for patients and change the day-to-day workflow for clinicians. Embedding analytics, machine learning, and data-driven quality improvement capabilities within the tools are natural when these specialties are deeply ingrained as part of the software development.
References: